-
- 錯誤率:分錯樣本佔樣本總數的比例
- 精度:分對樣本佔樣本總數的比率
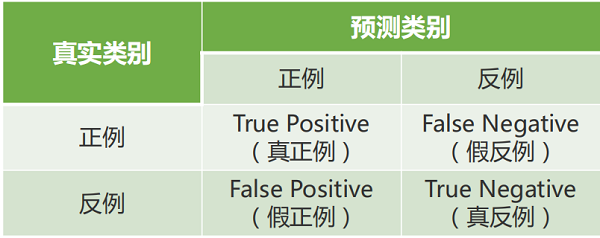
準確率 (Accuracy )= $\frac{T P+T N}{T P+T N+F P+F N}$ 預 測 正 確 的 結 果 佔 總 樣 本 的 百 分 比
查準率 ( Precision ) = $\frac{T P}{TP+F P}$ 挑出的西瓜中有多少比例是好的?
查全率/召回率/敏感度 ( Recall/Sensitivity ) = $\frac{T P}{T P+F N} $
- 若一個學習演算法的PR曲線被另一個學習演算法的曲線完全“包住”,則可認為後者的效能優於前者,如A優於C;
- 若兩個學習演算法的PR曲線發生交叉(如A和B),則難以判斷孰優孰劣,只能在具體的查準率和查全率條件下進行比較;
- 可通過比較P-R曲線下的面積(PR-AUC)
- 利用平衡點(即P=R時的取值)
- 利用F1度量
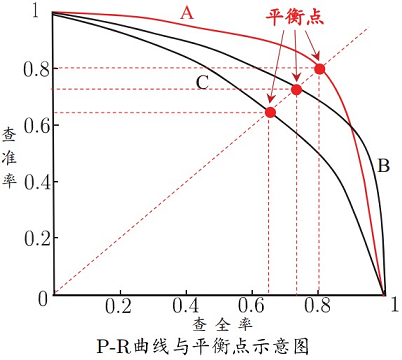
$ \beta=1$: 標準F1
$\beta>1 $: 偏重查全率(逃犯資訊檢索)
$ \beta<1$ : 偏重查準率(商品推薦系統)
我們希望在 $ \mathrm{n} $ 個二分類混淆矩陣上綜合考慮查準率和查全率
先在混淆矩陣上分別計算出查準率和查全率,記為 $\left(P_{1}, R_{1}\right),\left(P_{2}, R_{2}\right), \ldots,\left(P_{n}, R_{n}\right) $, 再計算平均值,這樣得到“巨集查準率”、“巨集查全率”以及“巨集 F1 ” :
$\begin{aligned}\text { macro }-\boldsymbol{P} &=\frac{1}{n} \sum \limits _{i=1}^{n} P_{i} \\\text { macro }-\boldsymbol{R} &=\frac{1}{n} \sum \limits _{i=1}^{n} R_{i} \\\text { macro }-\boldsymbol{F} \mathbf{1} &=\frac{2 \times \text { macro }-P \times \text { macro }-R}{\text { macro }-P+\text { macro }-R}\end{aligned}$
- l Macro-averaged gives equal weight to each class
- l Micro-averaged gives equal weight to each per-instance classification decision
- l Macro-averaging is a good choice when you get a sense of effectiveness on small classes
- l Micro-averaging is a good choice on the large classes because large classes dominate small classes in micro-averaging
- l Macro-averaging evaluates the system performance overall across the sets of data, can not get any specific decision with it
- l Micro-average can be a useful measure when the dataset varies in size
真正率 (TPR ) = 靈敏度/召回率 = $\mathrm{TP} /(\mathrm{TP}+\mathrm{FN}) $ 正例中有多少樣本被檢測出
假正率 (FPR ) = 1- 特異度 = $\mathrm{FP} /(\mathrm{FP}+\mathrm{TN}) $ 負例中有多少樣本被錯誤覆蓋
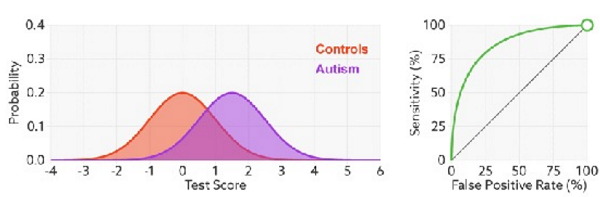
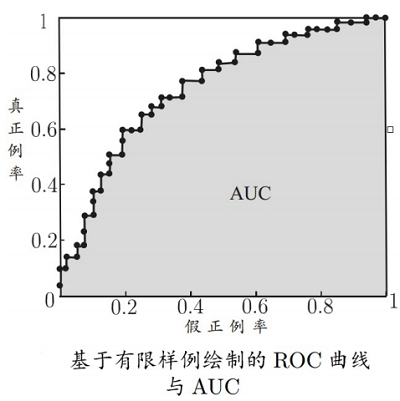
假設ROC曲線由 $\left\{\left(x_{i}, y_{i}\right)\right\}_{i=1}^{m}$ 的點按序連線而 形成 $\left(x_{1}=0, x_{m}=1\right)$ 則 $A \cup C$ 可估算為 :
$\mathrm{AUC}=\frac{1}{2} \sum_{i=1}^{m-1}\left(x_{i+1}-x_{i}\right) \cdot\left(y_{i}+y_{i+1}\right)$
AUC衡量了樣本預測的排序質量。
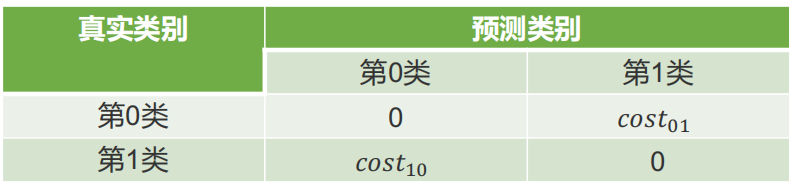
在非均等代價下,不再最小化錯誤次數,而是最小化“總體代價”,則“代價敏感”錯誤率相應的為:
$P(+) \operatorname{cost}=\frac{p \times \cos t_{01}}{p \times \operatorname{cost}_{01}+(1-p) \times \operatorname{cost}_{10}}$
縱軸是取值為 $[0,1] $ 的歸一化代價
$\operatorname{cost}_{n o r m}=\frac{\text { FNR } \times p \times \operatorname{cost}_{01}+\text { FPR } \times(1-p) \times \operatorname{cost}_{10}}{p \times \operatorname{cost}_{01}+(1-p) \times \operatorname{cost}_{10}}$
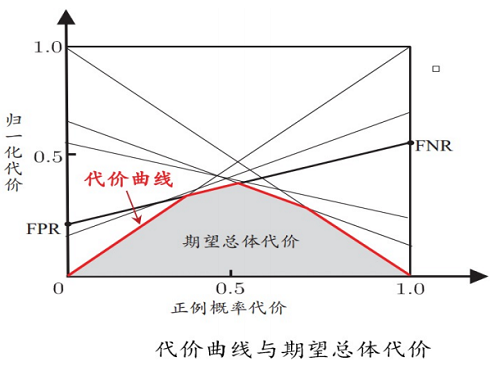
